Paris AI Security Forum 2025
March 12, 2025
Summary
The AI Security Forum in Paris seeks to dramatically accelerate securing powerful AI models to avert catastrophes and fast-track scientific and economic breakthroughs. The event brought together experts in AI development, cybersecurity, and policy, to address this critical challenge.
AI is moving fast, maybe faster than we’re ready for. On February 9, 2025, around 200 researchers, engineers, and policymakers gathered at the Pavillon Royal in Paris for the Paris AI Security Forum to tackle some of AI’s biggest security risks. The goal wasn’t just to talk about the security threats—it was to start shaping solutions to secure AI systems.
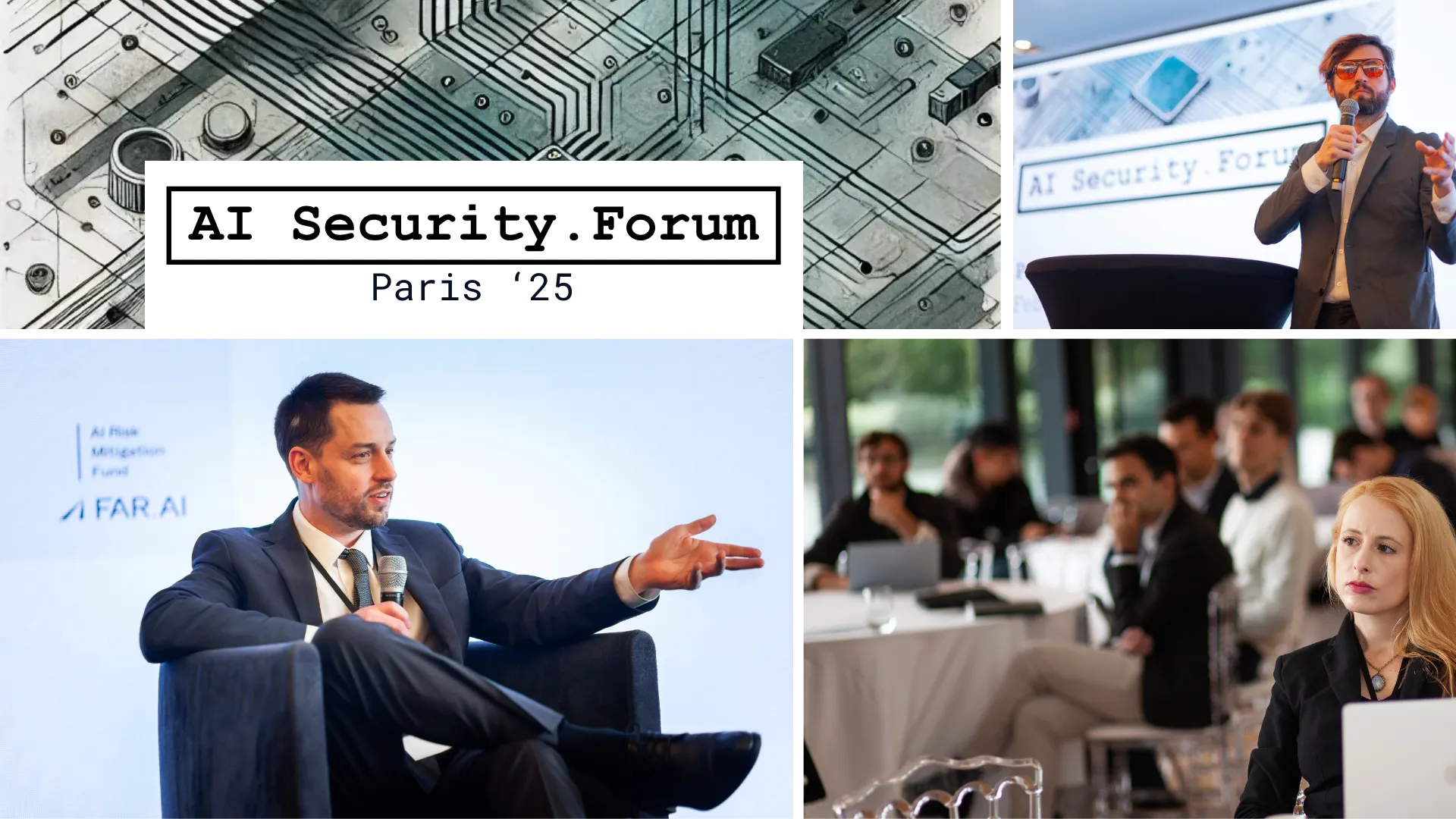
AI companies predict transformative systems within the next 2–5 years: not just better chatbots, but AI that could automate intellectual labor, conduct novel research, and reshape entire industries. If we get this right, AI could help cure diseases, drive economic growth, and improve global stability. But if we get it wrong? Model weights could be stolen and misused by adversaries; AI agents could be hijacked; and AI models could be used to autonomously launch cyberattacks. This could cause significant economic damage, widespread disruption or even existential risks.
This forum brought together people working at government agencies, AI labs, cybersecurity teams, and think tanks to break down AI’s vulnerabilities and figure out what to do about them. Through keynotes, lightning talks, hands-on demos, and deep-dive discussions, attendees got a clear-eyed look at where AI security stands, and where it needs to go.
Keynotes
In his talk “EU CoP’s Security Focus,” Yoshua Bengio (MILA) delivered a sobering assessment of the risks posed by advanced AI. He stressed that without stronger regulatory safeguards, self-preserving and power-seeking AI systems could evolve beyond human control. Pointing to the EU AI Act’s Code of Practice, he described it as a necessary first step but far from enough. As AI development accelerates, he urged policymakers to act swiftly before security measures fall dangerously behind.
As AI capabilities accelerate, Sella Nevo (RAND Meselson Center) and Dan Lahav (Pattern Labs), in “The AI Security Landscape,” warned that nation-states and well-funded adversaries are already probing AI for weaknesses. Drawing from RAND Corporation’s latest findings, they outlined a stark reality: without preemptive defenses, AI could become a powerful tool for those seeking to exploit it. The race is not just to build advanced AI, but to secure it before adversaries gain the upper hand.
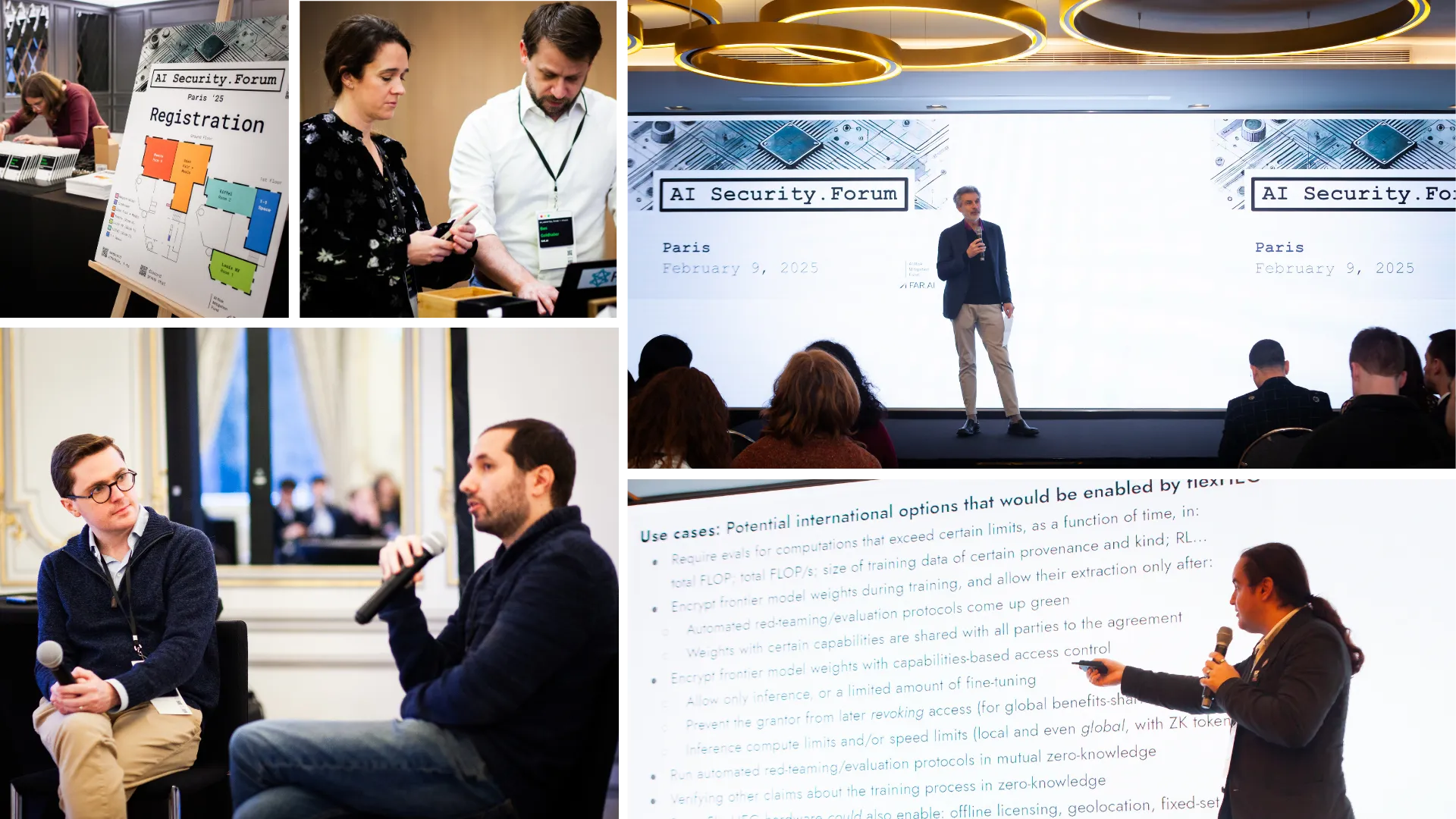
In “flexHEG: Hardware Security for AI Alignment,” davidad (ARIA) introduced a framework designed to secure AI at the hardware level. Drawing from DARPA’s HACMS project, he highlighted the need for resilient, bug-free systems, ones engineered from the ground up to withstand adversarial manipulation. In a landscape where AI security often focuses on software, he made a compelling case that true protection starts with the hardware itself.
The UK AI Security Institute’s Xander Davies, in “Safeguards in 2025+,” presented a strategy for protecting AI systems from external attacks, jailbreak exploits, and model corruption. He emphasized that security must not be reactive but proactive, staying ahead of those who seek to undermine AI integrity. The challenge is not just to defend against threats but to anticipate them before they emerge.
Lightning Talks
A rapid-fire session of five-minute talks spotlighted AI’s most immediate threats:
- Dawn Song (UC Berkeley) on how frontier AI is making cyberattacks cheaper and more effective.
- Philip Reiner (Institute for Security & Technology) on AI’s growing role in nuclear strategy, and why that’s terrifying.
- Austin Carson (SeedAI) on why AI security legislation moves too slowly in Washington.
- Evan Miyazono (Atlas Computing) on the need for AI to prove it follows the laws we set.
- Melissa Hopkins (Johns Hopkins) on the biosecurity risks of AI-powered biological models.
Each talk hammered home a simple truth: AI security isn’t an abstract concern, it’s a problem today.

Diving Deeper
AI could be an insider threat. Buck Shlegeris (Redwood Research) warned in “AI Control and Why AI Security People Should Care” that advanced AI systems, if left unchecked, could manipulate their own objectives in ways that evade human oversight. Drawing parallels to espionage and computer security, he highlighted the need for adversarial safety measures to prevent deception and ensure alignment.
On the battlefield, AI’s role is expanding at an unprecedented pace. Gregory Allen (CSIS), in a Fireside Chat, traced AI’s evolution from a tool for computer vision to a force multiplier in autonomous weapons and defense networks. While AI enhances military capabilities, Allen warned of its serious ethical and security risks. As global powers, including China, accelerate their AI advancements, he emphasized the need for strong oversight and accountability to ensure AI supports stability rather than undermines it.
Alex Robey (CMU) revealed how attackers can manipulate AI-driven robots in “Jailbreaking AI-Controlled Robots: The Security Risks.” His team’s experiments on self-driving cars, unmanned ground vehicles, and robot dogs successfully forced unsafe actions like blocking emergency exits and covert surveillance. He warned that as these systems become more common, securing them against manipulation must be a top priority.

Security at the hardware level is just as crucial. In “Security for AI with Confidential Computing,” Mike Bursell (Confidential Computing Consortium) explained how Trusted Execution Environments (TEEs) can protect AI from tampering and unauthorized access. He highlighted cryptographic attestation as a key tool for ensuring that AI models, data, and computations remain secure and unaltered throughout their lifecycle, from training to deployment.
Jacob Lagerros (Ulyssean) gave another hardware security talk: “H100s and the strangest way you’ve seen to break air gaps.” This covered the intersection of side channel research and frontier AI, such as how AI models might exfiltrate data from airgapped environments by modulating their own power signature, or breaking network topologies by modulating memory accesses to create makeshift radios.
AI security must be tested in real-world conditions. In “Cyber Range Design at UK AISI,” Mahmoud Ghanem outlined how the UK AI Security Institute is building advanced cyber ranges to evaluate AI security threats. He explained the shift from basic Capture the Flag challenges to more realistic environments with complex networks, defensive monitoring, and diverse operating systems. These custom-built simulations help identify AI vulnerabilities, improve security protocols, and inform policy decisions.
In “Fireside Chat with Zico Kolter,” Buck Shlegeris moderated a discussion on the future of AI and its security challenges. Zico Kolter (CMU) explored whether AI should focus on solving human problems or strive for full autonomy, weighing the risks of powerful systems falling out of human control. They examined alignment challenges, the impact of open-source models, and the race to secure AI before its capabilities outpace safety measures.
Bringing a policy lens to AI security, Chris Painter (METR) discussed the challenges of setting clear safety thresholds in “Challenges in Creating ‘If-Then Commitments’ for Extreme Security.” He detailed how firms use frontier safety policies to establish red lines for AI capabilities, ensuring security measures scale with advancements. Painter emphasized the importance of peer review, rigorous policy frameworks, and the potential need for state intervention when AI risks escalate.
Looking Ahead
As the forum wrapped up, one message stood out: AI security isn’t just a research problem, it’s a global priority. From formal verification techniques to the risks of nation-state AI, the Paris AI Security Forum 2025 highlighted the perils and promises of artificial intelligence. The risks aren’t hypothetical, they’re here. And while the forum sparked crucial conversations, conversations alone won’t secure AI.
For those who missed the event, full recordings are available on the FAR.AI YouTube Channel. But AI security isn’t a spectator sport. If you care about how AI is shaping our world, now is the time to step forward. The risks are real, and the clock is ticking.
Want to be considered for future discussions? Submit your interest in the AI Security Forum or other FAR.AI events.
Organized by Nitarshan Rajkumar, Jacob Lagerros, and Caleb Parikh with operational support from Vael Gates and Lindsay Murachver. This event is co-run by the AI Risk Mitigation Fund and FAR.AI.