Abstract
Work on scaling laws has found that large language models (LMs) show predictable improvements to overall loss with increased scale (model size, training data, and compute). Here, we present evidence for the claim that LMs may show inverse scaling, or worse task performance with increased scale, e.g., due to flaws in the training objective and data. We present empirical evidence of inverse scaling on 11 datasets collected by running a public contest, the Inverse Scaling Prize, with a substantial prize pool. Through analysis of the datasets, along with other examples found in the literature, we identify four potential causes of inverse scaling: (i) preference to repeat memorized sequences over following in-context instructions, (ii) imitation of undesirable patterns in the training data, (iii) tasks containing an easy distractor task which LMs could focus on, rather than the harder real task, and (iv) correct but misleading few-shot demonstrations of the task. We release the winning datasets online to allow for further investigation of inverse scaling. Our tasks have helped drive the discovery of U-shaped and inverted-U scaling trends, where an initial trend reverses, suggesting that scaling trends are less reliable at predicting the behavior of larger-scale models than previously understood. Overall, our results suggest that there are tasks for which increased model scale alone may not lead to progress, and that more careful thought needs to go into the data and objectives for training language models.
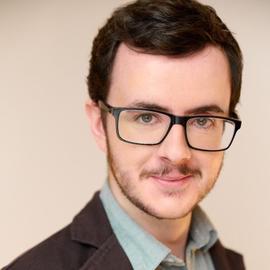
Research Engineer
Ian McKenzie is a research engineer at FAR.AI, where he previously ran the Inverse Scaling Prize.
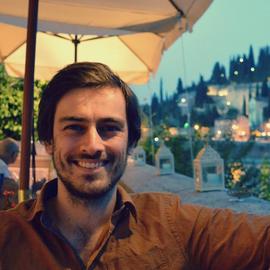
Communications Specialist
Euan is a communications specialist at FAR.AI. In the past he has completed a PhD in theoretical particle physics at the University of Glasgow, worked as a machine learning engineer at a cybersecurity startup, and worked as a strategy researcher at the Center on Long Term Risk. He is also a scriptwriter for the YouTube channel PBS Spacetime. His passion is reducing interpretive labor in AI alignment to speed up the progress of the field.
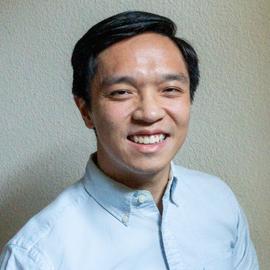
Research Engineer
Tom Tseng is a research engineer at FAR.AI. Tom previously worked as a software engineer at Gather and Cruise. He has a master’s degree from MIT and a bachelor’s degree from Carnegie Mellon University.
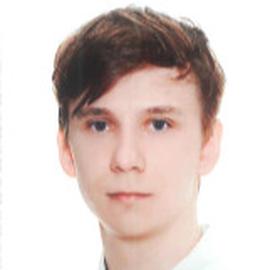
PhD Student
Tomas is a PhD student at the Department of Informatics, University of Sussex working on deep reinforcement learning and generative models with Chris Buckley and Anil Seth. He focuses on probabilistic approaches to control, such as active inference and control-as-inference, and controllable generative modelling. Tomas previously worked at FAR.AI with Ethan Perez and Sam Bowman on aligning language models with human preferences. For more information, see his website.
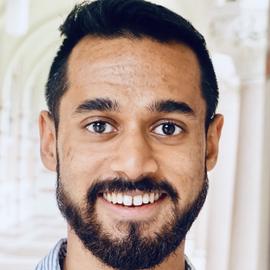
Research Scientist
Ethan is a Research Scientist at Anthropic. He completed his Ph.D. in Natural Language Processing at New York University. He was advised by Kyunghyun Cho and Douwe Kiela and funded by NSF and Open Philanthropy. His research focuses on aligning language models with human preferences, e.g., for content that is helpful, honest, and harmless. In particular, he is excited about developing learning algorithms that outdo humans at generating such content, by producing text that is free of social biases, cognitive biases, common misconceptions, and other limitations. Previously, he has spent time at DeepMind, Facebook AI Research, Montreal Institute for Learning Algorithms, Uber, and Google. He earned a Bachelor’s from Rice University as the Engineering department’s Outstanding Senior. Visit his website to find out more.