Abstract
Language model capabilities predictably improve from scaling a model`s size and training data. Motivated by this, increasingly large language models have been trained, yielding an array of impressive capabilities. Yet these models are vulnerable to adversarial prompts, such as “jailbreaks” that hijack models to perform undesired behaviors, posing a significant risk of misuse. Prior work indicates that computer vision models become more robust with model and data scaling, raising the question: does language model robustness also improve with scale? We study this question empirically, finding that larger models respond substantially better to adversarial training, but there is little to no benefit from model scale in the absence of explicit defenses.
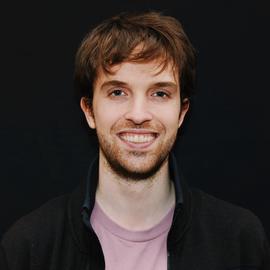
PhD Candidate
Niki Howe is a PhD candidate at Mila and the Université de Montréal, where they also received their MSc. They hold BAs in Math and CS from Williams College and the University of Cambridge, respectively. At FAR.AI, Niki is exploring how scale affects the robustness of LLMs.
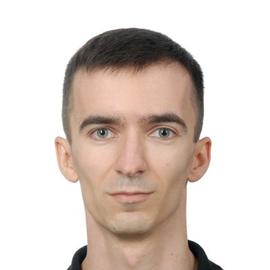
Research Engineer
Michał Zając is a research engineer at FAR.AI. Prior to joining FAR.AI, he completed a PhD on deep reinforcement learning at Jagiellonian University, and has worked as an engineer at Allegro, Google and Nomagic.
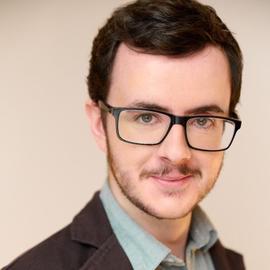
Research Engineer
Ian McKenzie is a research engineer at FAR.AI, where he previously ran the Inverse Scaling Prize.
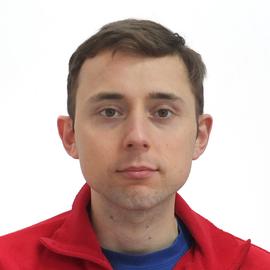
Research Resident
Oskar Hollinsworth is a research resident at FAR.AI, working on scaling laws for adversarial robustness.
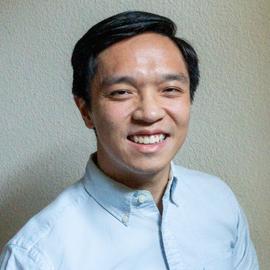
Research Engineer
Tom Tseng is a research engineer at FAR.AI. Tom previously worked as a software engineer at Gather and Cruise. He has a master’s degree from MIT and a bachelor’s degree from Carnegie Mellon University.
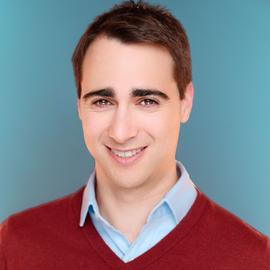
CEO and President of the Board
Adam Gleave is the CEO of FAR.AI. He completed his PhD in artificial intelligence (AI) at UC Berkeley, advised by Stuart Russell. His goal is to develop techniques necessary for advanced automated systems to verifiably act according to human preferences, even in situations unanticipated by their designer. He is particularly interested in improving methods for value learning, and robustness of deep RL. For more information, visit his website.