Bay Area Alignment Workshop 2024
Website YouTube Express Interest
On October 24-25, 2024, Santa Cruz became the focal point for AI safety as 160 researchers and leaders from academia, industry, government, and nonprofits gathered for the Bay Area Alignment Workshop. Against a backdrop of pressing concerns around advanced AI risks, attendees convened to guide the future of AI toward safety and alignment with societal values.
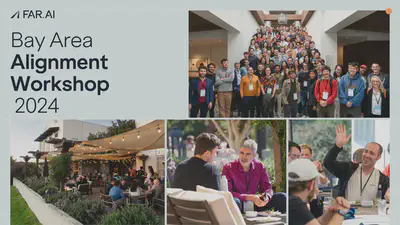
Over two packed days, participants engaged with pivotal themes such as evaluation, robustness, interpretability and governance. The workshop unfolded across multiple tracks and lightning talks, enabling in-depth exploration of topics. Diverse participants from industry labs, academia, non-profits and governments shared insights into their organizations’ safety practices and latest discoveries. Each evening featured open dialogues ranging from the sufficiency of current safety portfolios to a fireside chat on the Postmortem of SB 1047. The collaborative atmosphere fostered lively debate and networking, creating a vital platform for discussing—and shaping—the critical safeguards needed as AI systems advance.
Introduction & Threat Models: Optimized Misalignment
Anca Dragan, Director of AI Safety and Alignment at Google DeepMind, kicked off the event with Optimized Misalignment, highlighting the risk of advanced AI systems pursuing goals misaligned with human values due to flawed reward models. She urged the AI community to adopt robust threat modeling practices, emphasizing that as AI power grows, managing these misalignment risks is critical for safe development.
Monitoring & Assurance
In METR Updates & Research Directions, Beth Barnes emphasized the need for rigorous evaluation methods that measure and forecast risks in advanced AI. While noting progress in R&D contexts, Barnes stressed that improved elicitation is essential for accurate assessment. She advocated for open-source evaluations to enhance transparency and foster collaboration across frontier model developers.
Buck Shlegeris of Redwood delivered AI Control: Strategies for Mitigating Catastrophic Misalignment Risk, exploring techniques like trusted monitoring, collusion detection, and adversarial testing to manage potentially misaligned goals in AI. Shlegeris likened these protocols to insider threat management, arguing that robust safety practices are both achievable and necessary for securing powerful AI models.
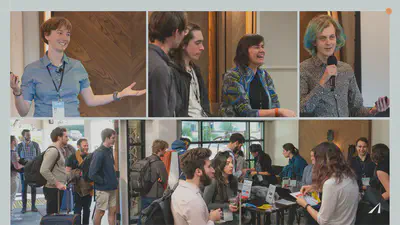
Governance & Security
Moderated by Gillian Hadfield, the Governance & Security session presented global perspectives on AI policy. Hamza Chaudhry offered updates from Washington, D.C. Nitarshan Rajkumar provided an overview of the UK’s AI Strategy, while Siméon Campos discussed the EU AI Act & Safety. Sella Nevo shared perspectives on AI security.
Kwan Yee Ng presented AI Policy in China, drawing from Concordia AI’s recent report. Ng described China’s binding safety regulations and regional pilot programs in cities like Beijing and Shanghai, which aim to address both immediate and long-term AI risks. She underscored China’s approach to AI safety as a national priority, framing it as a public safety and security issue.
Interpretability
Atticus Geiger’s talk, State of Interpretability & Ideas for Scaling Up, focused on methods for predicting, controlling, and understanding models. Geiger critiqued current approaches like sparse autoencoders (SAEs), advocating instead for causal abstraction to map model behaviors to human-understandable algorithms, positioning interpretability as essential to AI safety.
On Improving AI Safety with Top-Down Interpretability, Andy Zou presented a “top-down” approach inspired by cognitive neuroscience, which centers on understanding global model behaviors rather than individual neurons. Zou demonstrated how this perspective could help control emergent properties like honesty and adversarial resistance, ultimately enhancing model safety.
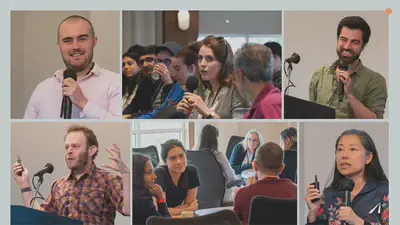
Robustness
Stephen Casper’s talk, Powering Up Capability Evaluations, highlighted the need for rigorous third-party evaluations to guide science-based AI policy. He argued that model manipulation attacks reveal vulnerabilities missed by standard tests, especially in open-weight models, with LORA fine-tuning an effective stress-testing method.
Alex Wei, in Paradigms and Robustness, advocated for reasoning-based approaches to improve model resilience. He suggested that allowing AI models to “reason” before generating responses could help prevent issues like adversarial attacks and jailbreaks, offering a promising path forward for model robustness.
Adam Gleave’s talk, Will Scaling Solve Robustness? questioned whether simply scaling models increases resilience. He discussed the limitations of adversarial training and called for more efficient solutions to match AI’s growing capabilities without compromising safety.
Oversight
Micah Carroll’s talk, Targeted Manipulation & Deception Emerge in LLMs Trained on User Feedback, revealed concerning behaviors in language models optimized for user feedback, such as selectively deceiving users based on detected traits. Carroll called for oversight methods that prevent such manipulation without making deceptive behaviors subtler and harder to detect.
Julian Michael, in Empirical Progress on Debate, explored debate as a scalable oversight tool, particularly for complex, high-stakes tasks. By setting AIs against each other in structured arguments, debate protocols aim to enhance human judgment accuracy. Michael introduced “specification sandwiching” as a method to align AI more closely with human intent, reducing manipulative tendencies.
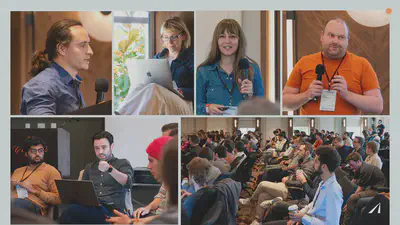
Lightning Talks
Day 1 lightning talks covered diverse topics spanning Agents, Alignment, Interpretability, and Robustness. Daniel Kang discussed the dual-use nature of AI agents. Kimin Lee introduced MobileSafetyBench, a tool for evaluating autonomous agents in mobile contexts. Sheila McIlraith encouraged using formal languages to encode reward functions, instructions, and norms. Atoosa Kasirzadeh examined AI alignment within value pluralism frameworks. Chirag Agarwal raised concerns about the reliability of chain-of-thought reasoning. Alex Turner presented gradient routing techniques for localizing neural computations. Jacob Hilton used backdoors as an analogy for deceptive alignment. Mantas Mazeika proposed tamper-resistant safeguards for open-weight models. Zac Hatfield-Dodds critiqued formal verification. Evan Hubinger shared insights from alignment stress-testing at Anthropic.
On day 2, the lightning talks shifted focus to Governance, Evaluation, and other high-level topics. Richard Ngo reframed AGI threat models. Dawn Song advocated for a sociotechnical approach to responsible AI development. Shayne Longpre introduced the concept of a safe harbor for AI evaluation and red teaming. Soroush Pour shared third-party evaluation insights from Harmony Intelligence. Joel Leibo presented on AGI-complete evaluation. David Duvenaud discussed linking capability evaluations to danger thresholds for large-scale deployments.
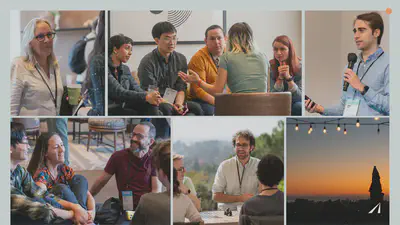
Impacts & Future Directions
The Bay Area Alignment Workshop advanced critical conversations on AI safety, fostering a stronger community committed to aligning AI with human values. To watch the full recordings, please visit our website or YouTube channel. If you’d like to attend future Alignment Workshops, register your interest here.
Website YouTube Express Interest
Special thanks to our Program Committee:
- Anca Dragan – Director, AI Safety and Alignment, Google DeepMind; Associate Professor, UC Berkeley
- Robert Trager – Co-Director, Oxford Martin AI Governance Initiative
- Dawn Song – Professor, UC Berkeley
- Dylan Hadfield-Menell – Assistant Professor, MIT
- Adam Gleave – Founder, FAR.AI