NOLA Alignment Workshop 2023
February 7, 2024
Summary
The New Orleans Alignment Workshop 2023 brought together leading ML researchers working to align advanced AI with human values and develop safe AI system. Presentations from industry, academia, and non-profits focused on topics spanning from oversight, interpretability, robustness, generalization and governance.
The New Orleans (NOLA) Alignment Workshop held on December 10-11, 2023 immediately prior to NeurIPS, brought together leading researchers working to align advanced AI systems with human values and develop safe AI systems. Hosted by FAR AI, the event drew 149 participants, featured a keynote by Turing Award laureate Yoshua Bengio, 12 insightful presentations, and 25 lightning talks. An evening social event added a festive touch, attracting over 500 guests.
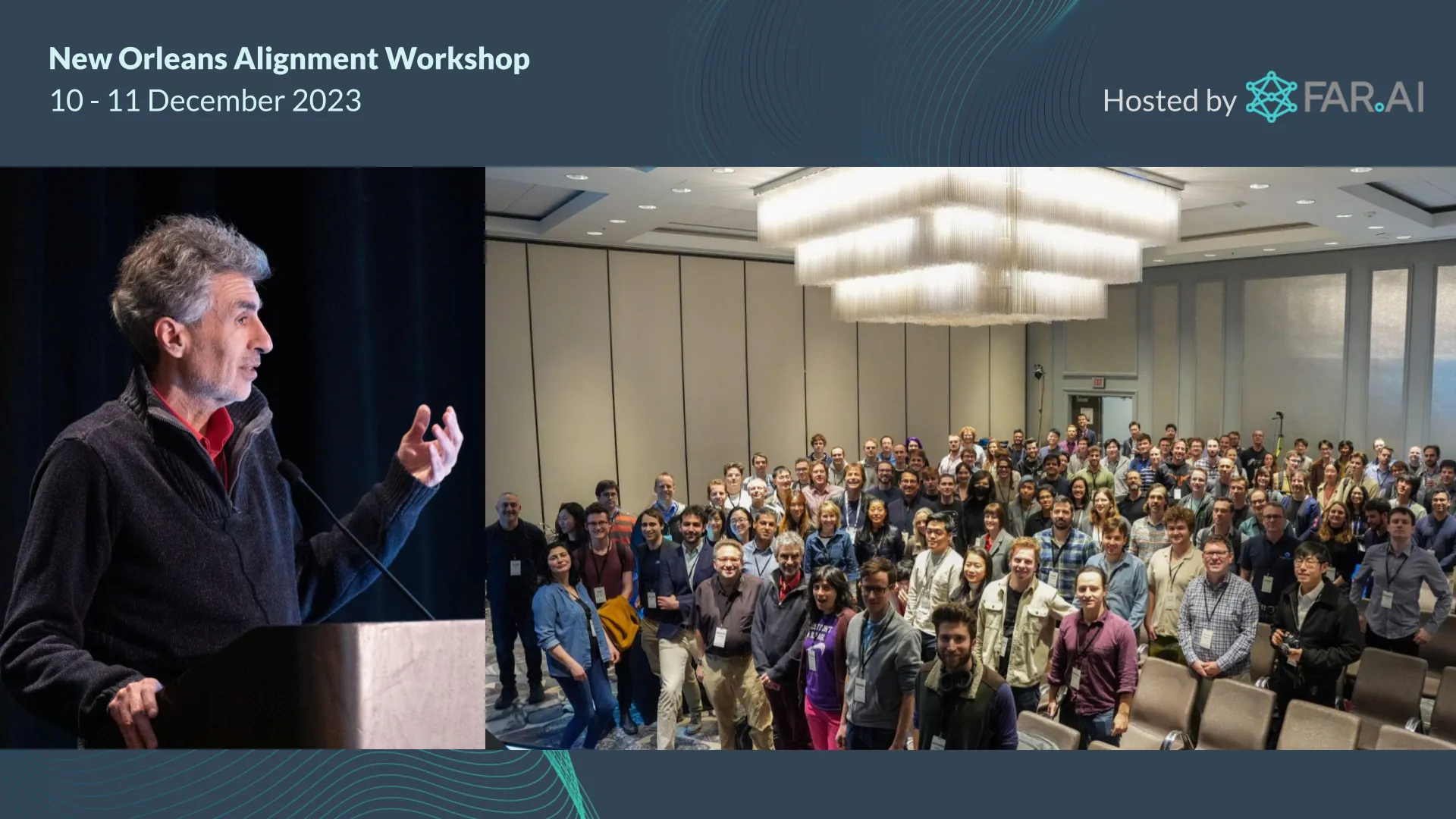
The workshop served as a hub for exchanging ideas, with attendees hailing from industry giants like OpenAI, Google DeepMind, and Anthropic, alongside academic institutions such as UC Berkeley, MIT, and Mila. Members from non-profits like the Center for AI Safety and the Cooperative AI Foundation, and various government agencies, further diversified the discussion. The central focus was on uniting the global AI alignment community to better understand AI risks, connect different research interests, and build upon the progress of previous workshops.
Keynote and Introducing Alignment Problems
In the opening remarks, Richard Ngo articulated the workshop's goals: to bridge diverse approaches in addressing AI risks and focus on concrete research directions. Tim Lillicrap then took the stage, sharing his personal experiences, underscoring a sense of urgency, and emphasizing the need for open-minded collaborations to develop effective solutions for AI safety and alignment.
Yoshua Bengio's keynote, "Towards Quantitative Safety Guarantees and Alignment," set an inspirational tone for the event, emphasizing the need for global, coordinated AI governance rooted in democratic values. He delved into the application of Bayesian methods for AI Alignment, highlighting the potential of GFlowNets in Bayesian structure learning, and advocating for a network of democratically governed AI labs to manage the challenges of AI advancements.
Adam Gleave's presentation, "AGI Safety: Risks and Research Directions," traced the evolution of AGI from the ideas of Turing and Minsky to today's urgent realities. He discussed the large-scale risks of AI misuse and rogue behavior, emphasizing the importance of Oversight, Robustness, Interpretability, and Governance in AI safety research.
Owain Evans, in his talk on "Out-of-context Reasoning in LLMs," highlighted potential risks from out-of-context reasoning enabling future models to “cheat” evaluations. Fortunately, currently even advanced models like GPT-4 struggle with complex out-of-context reasoning, however careful evaluation will be required for future models to detect this potentially dangerous capability.
Overall the talks covered an impressive range of topics, delving into various facets of AI alignment.
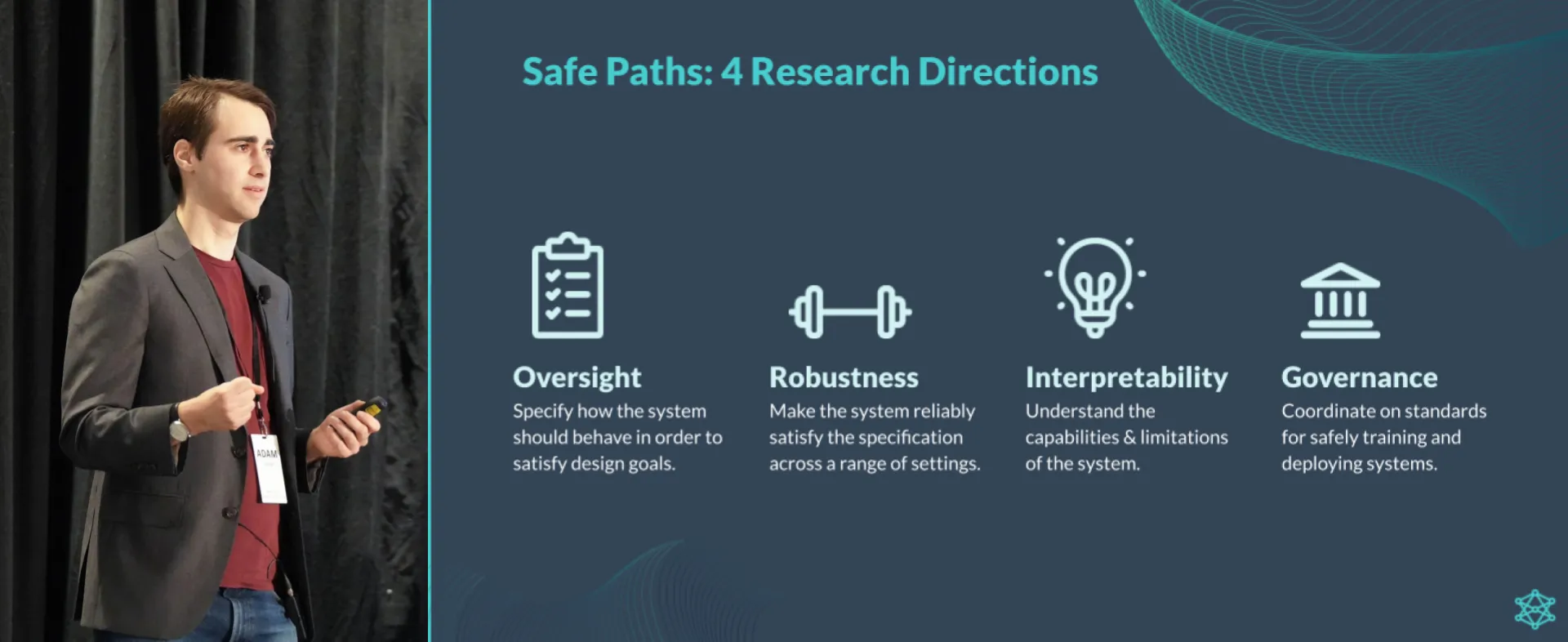
Oversight & Interpretability
Shifting the focus, Sam Bowman introduced "Adversarial Scalable Oversight for Truthfulness," emphasizing the importance of dependable AI in critical areas like disease research and complex scientific analysis. He delved into the concept of AI debates, where models argue both sides of a question to aid human judges in finding the most evidence-based answer. Tests indicate debates lead to more accurate conclusions, although limiting the debate complexity and length remains a challenge.
Meanwhile, Been Kim’s talk on "Alignment and Interpretability: How we might get it right," used the Korean concept of 'jeong' to illustrate the complexities in aligning human and machine understanding of a concept. She further explored AlphaGo's strategies and AlphaZero's influence on chess to demonstrate AI's potential to augment human expertise.
Another enlightening presentation was Roger Grosse's "Studying LLM Generalization through Influence Functions," where he explored how influence functions provide a novel approach to interpretability in LLMs by identifying what parts of the training data had the greatest influence on a given output. He moreover revealed LLMs growing ability to generalize in complex tasks like math and role-playing scenarios, underscoring the importance of integrating interpretability into AI research for a deeper understanding of AI learning patterns.

Robustness, Generalization, and Governance
Zico Kolter's live demonstration during "Adversarial Attacks on Aligned Language Models" was a standout moment, captivating the audience with his audacious and brilliant display of "jailbreaking" GPT-3.5 to hotwire a car, an act that underscored AI's immense power and vulnerabilities, emphasizing the need for robust alignment and safety measures. Collin Burns, in his talk on "Weak-to-Strong Generalization," delved into OpenAI's experiments using smaller AI models to supervise larger ones, highlighting a new frontier in AI alignment.
Meanwhile, in the Governance session, Gillian Hadfield’s talk, "Building an Off Switch for AI," proposed strategic regulation and a national AI registry, challenging the myth of AI's inevitable growth and advocating for a more responsible future in AI development, emphasizing the need for a robust legal infrastructure and economic incentives. Lightning talks explored a complex systems view, multi-agent risks, foundations of cooperative AI, and how to keep humans in the loop.
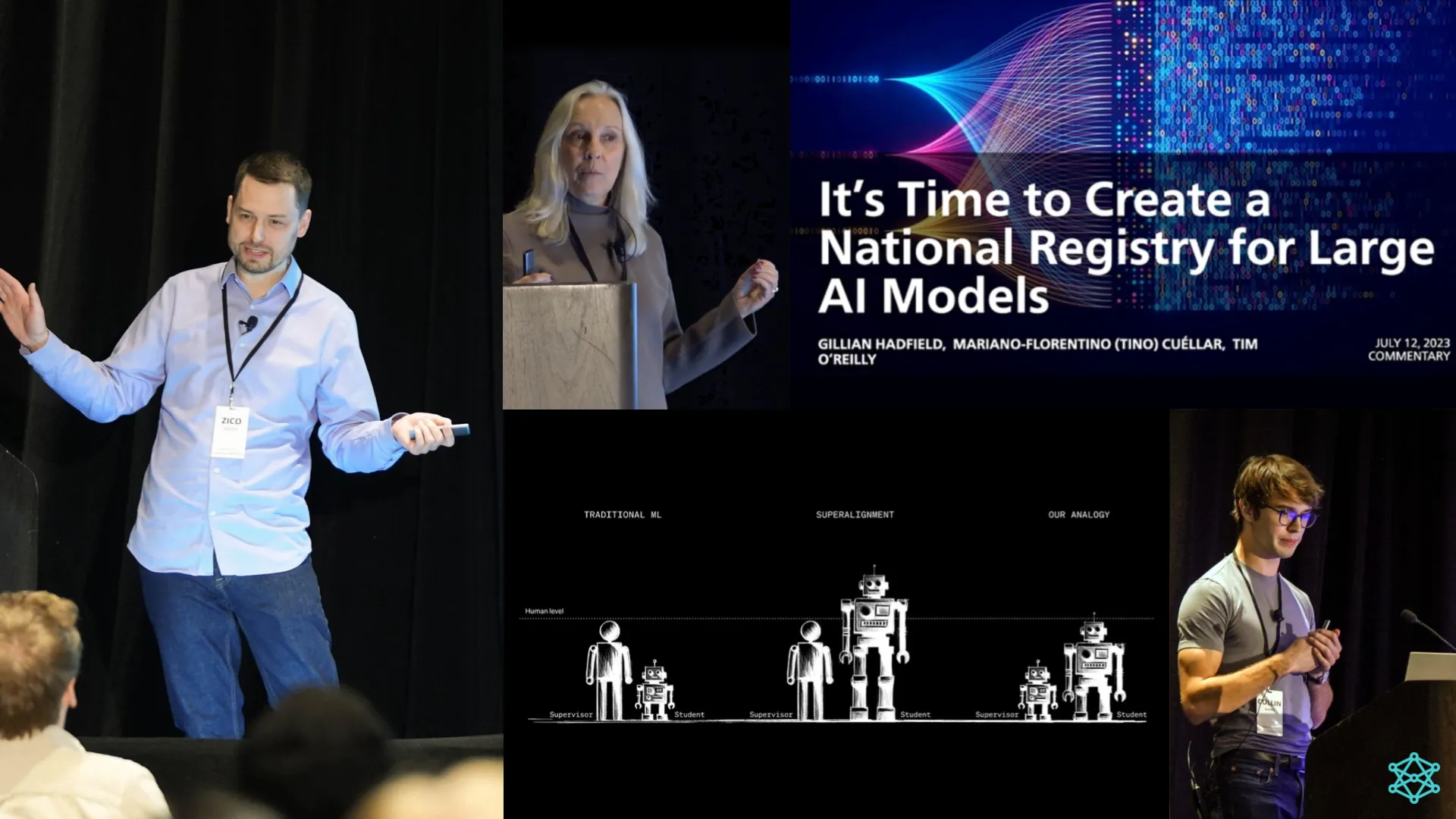
Impacts & Future Directions
The NOLA 2023 Alignment Workshop was more than just a gathering of minds; it served as a catalyst that brought AI alignment into the mainstream. The high-level participation and positive feedback from attendees, spanning industry labs, academia, non-profits, and government, underscored the community's readiness to tackle the challenges of advanced AI. Characterized by its high-quality presentations, collaborative spirit, and proactive discussions, the workshop set a new standard for future AI alignment events. As the AI community looks ahead, the insights and collaborations fostered at this event are set to play a crucial role in shaping a future where AI is not only advanced but also aligned with the greater good.

For the full recordings, please visit our website or YouTube channel. If you’d like to attend future Alignment Workshops, please register your interest in this short form.
This is a div block with a Webflow interaction that will be triggered when the heading is in the view.
The New Orleans (NOLA) Alignment Workshop held on December 10-11, 2023 immediately prior to NeurIPS, brought together leading researchers working to align advanced AI systems with human values and develop safe AI systems. Hosted by FAR AI, the event drew 149 participants, featured a keynote by Turing Award laureate Yoshua Bengio, 12 insightful presentations, and 25 lightning talks. An evening social event added a festive touch, attracting over 500 guests.
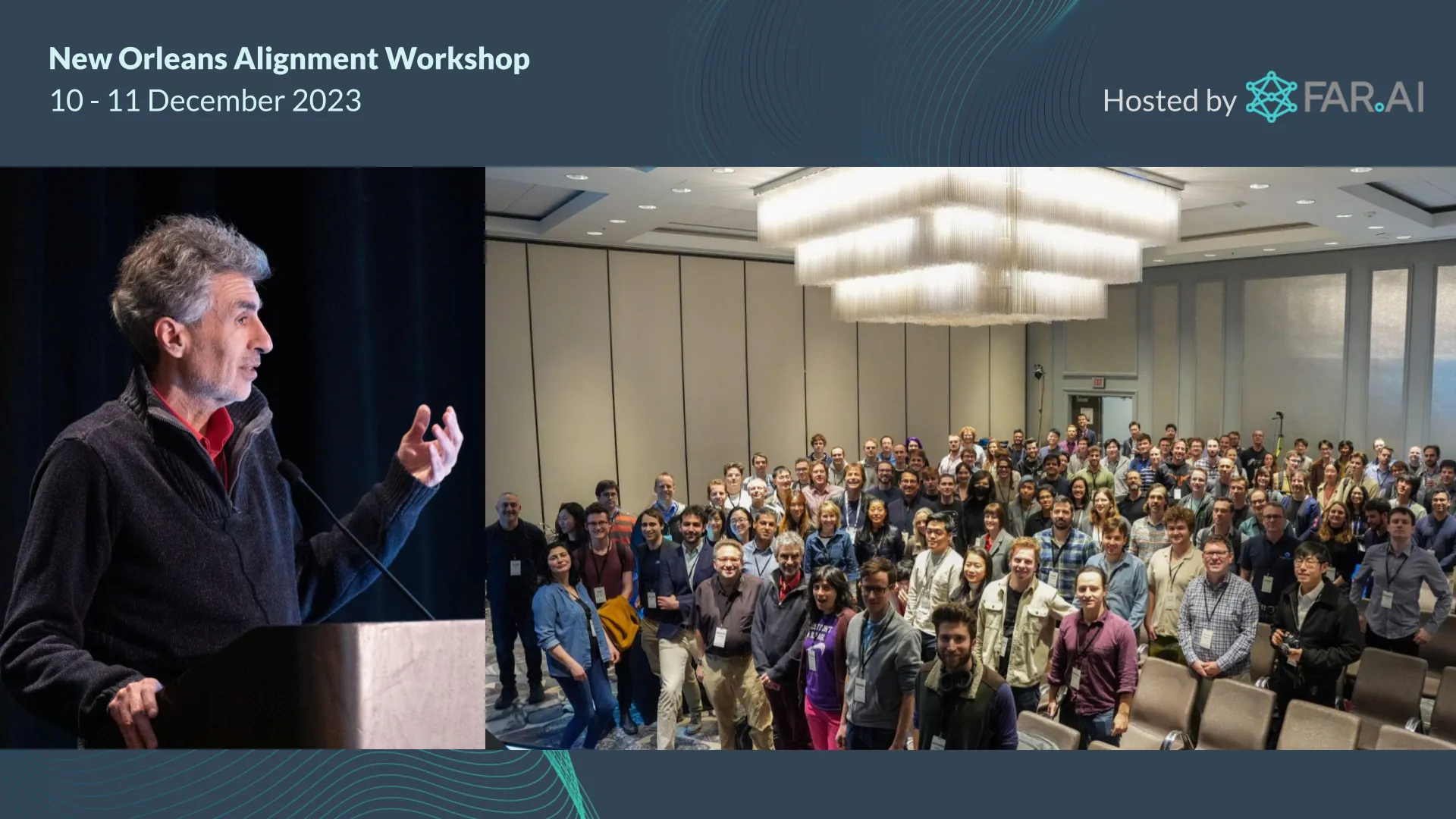
The workshop served as a hub for exchanging ideas, with attendees hailing from industry giants like OpenAI, Google DeepMind, and Anthropic, alongside academic institutions such as UC Berkeley, MIT, and Mila. Members from non-profits like the Center for AI Safety and the Cooperative AI Foundation, and various government agencies, further diversified the discussion. The central focus was on uniting the global AI alignment community to better understand AI risks, connect different research interests, and build upon the progress of previous workshops.
Keynote and Introducing Alignment Problems
In the opening remarks, Richard Ngo articulated the workshop's goals: to bridge diverse approaches in addressing AI risks and focus on concrete research directions. Tim Lillicrap then took the stage, sharing his personal experiences, underscoring a sense of urgency, and emphasizing the need for open-minded collaborations to develop effective solutions for AI safety and alignment.
Yoshua Bengio's keynote, "Towards Quantitative Safety Guarantees and Alignment," set an inspirational tone for the event, emphasizing the need for global, coordinated AI governance rooted in democratic values. He delved into the application of Bayesian methods for AI Alignment, highlighting the potential of GFlowNets in Bayesian structure learning, and advocating for a network of democratically governed AI labs to manage the challenges of AI advancements.
Adam Gleave's presentation, "AGI Safety: Risks and Research Directions," traced the evolution of AGI from the ideas of Turing and Minsky to today's urgent realities. He discussed the large-scale risks of AI misuse and rogue behavior, emphasizing the importance of Oversight, Robustness, Interpretability, and Governance in AI safety research.
Owain Evans, in his talk on "Out-of-context Reasoning in LLMs," highlighted potential risks from out-of-context reasoning enabling future models to “cheat” evaluations. Fortunately, currently even advanced models like GPT-4 struggle with complex out-of-context reasoning, however careful evaluation will be required for future models to detect this potentially dangerous capability.
Overall the talks covered an impressive range of topics, delving into various facets of AI alignment.
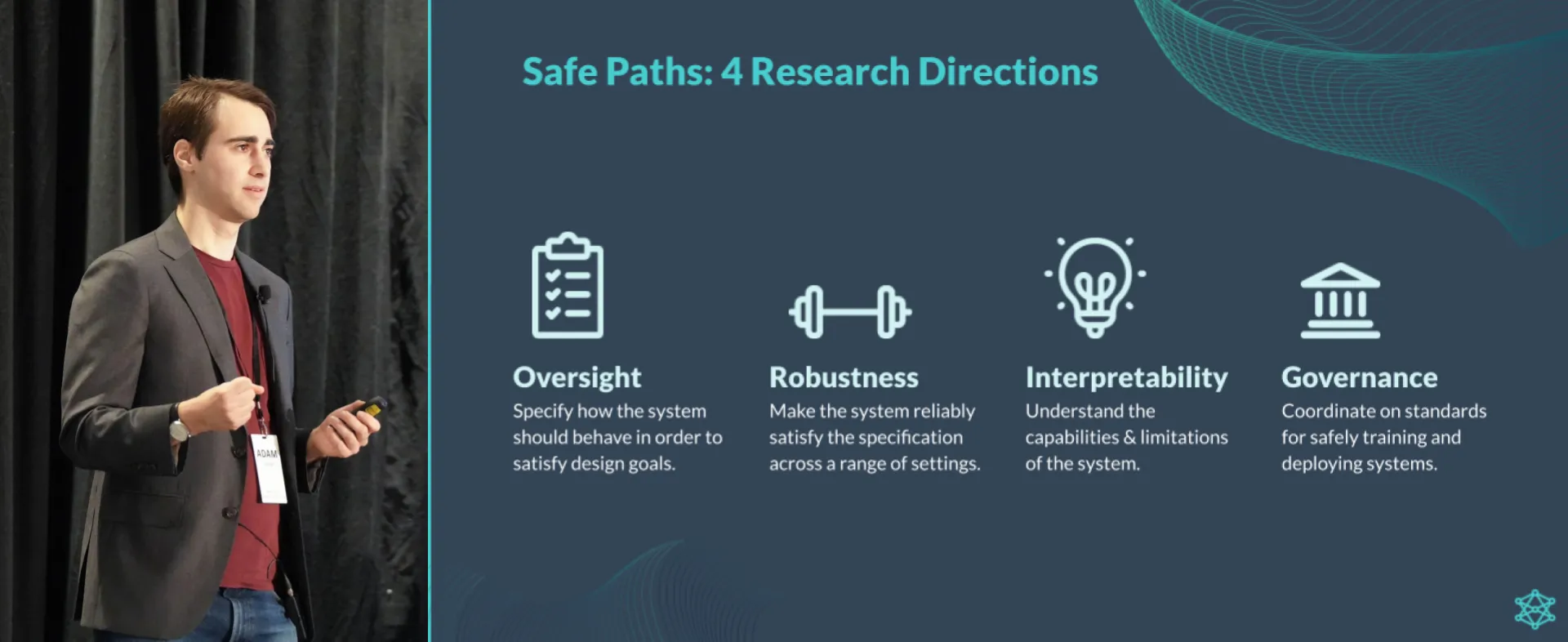
Oversight & Interpretability
Shifting the focus, Sam Bowman introduced "Adversarial Scalable Oversight for Truthfulness," emphasizing the importance of dependable AI in critical areas like disease research and complex scientific analysis. He delved into the concept of AI debates, where models argue both sides of a question to aid human judges in finding the most evidence-based answer. Tests indicate debates lead to more accurate conclusions, although limiting the debate complexity and length remains a challenge.
Meanwhile, Been Kim’s talk on "Alignment and Interpretability: How we might get it right," used the Korean concept of 'jeong' to illustrate the complexities in aligning human and machine understanding of a concept. She further explored AlphaGo's strategies and AlphaZero's influence on chess to demonstrate AI's potential to augment human expertise.
Another enlightening presentation was Roger Grosse's "Studying LLM Generalization through Influence Functions," where he explored how influence functions provide a novel approach to interpretability in LLMs by identifying what parts of the training data had the greatest influence on a given output. He moreover revealed LLMs growing ability to generalize in complex tasks like math and role-playing scenarios, underscoring the importance of integrating interpretability into AI research for a deeper understanding of AI learning patterns.

Robustness, Generalization, and Governance
Zico Kolter's live demonstration during "Adversarial Attacks on Aligned Language Models" was a standout moment, captivating the audience with his audacious and brilliant display of "jailbreaking" GPT-3.5 to hotwire a car, an act that underscored AI's immense power and vulnerabilities, emphasizing the need for robust alignment and safety measures. Collin Burns, in his talk on "Weak-to-Strong Generalization," delved into OpenAI's experiments using smaller AI models to supervise larger ones, highlighting a new frontier in AI alignment.
Meanwhile, in the Governance session, Gillian Hadfield’s talk, "Building an Off Switch for AI," proposed strategic regulation and a national AI registry, challenging the myth of AI's inevitable growth and advocating for a more responsible future in AI development, emphasizing the need for a robust legal infrastructure and economic incentives. Lightning talks explored a complex systems view, multi-agent risks, foundations of cooperative AI, and how to keep humans in the loop.
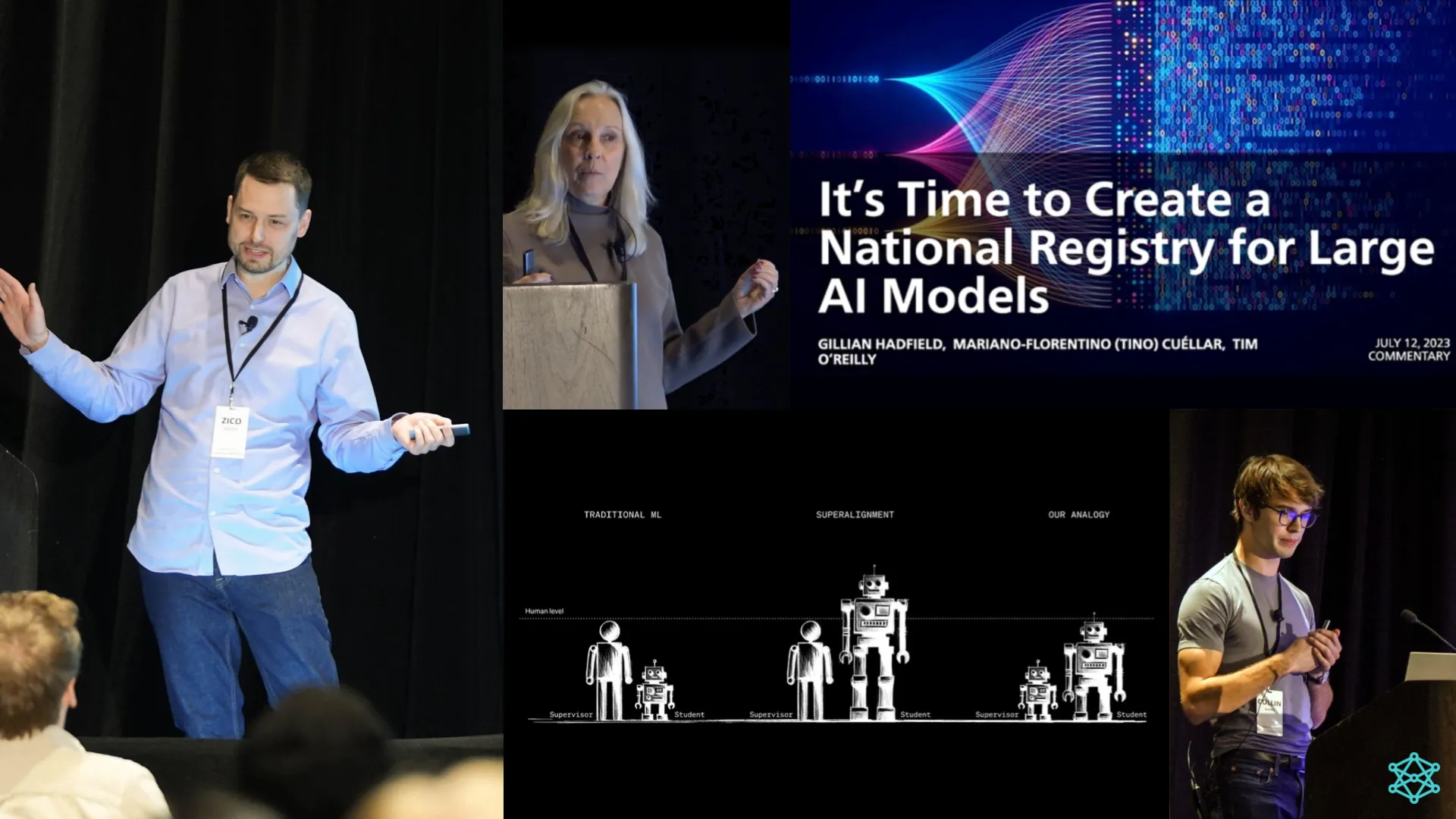
Impacts & Future Directions
The NOLA 2023 Alignment Workshop was more than just a gathering of minds; it served as a catalyst that brought AI alignment into the mainstream. The high-level participation and positive feedback from attendees, spanning industry labs, academia, non-profits, and government, underscored the community's readiness to tackle the challenges of advanced AI. Characterized by its high-quality presentations, collaborative spirit, and proactive discussions, the workshop set a new standard for future AI alignment events. As the AI community looks ahead, the insights and collaborations fostered at this event are set to play a crucial role in shaping a future where AI is not only advanced but also aligned with the greater good.

For the full recordings, please visit our website or YouTube channel. If you’d like to attend future Alignment Workshops, please register your interest in this short form.